I am actively looking for a summer internship. Please feel free to reach out to me at shivam.grover@queensu.ca.
News
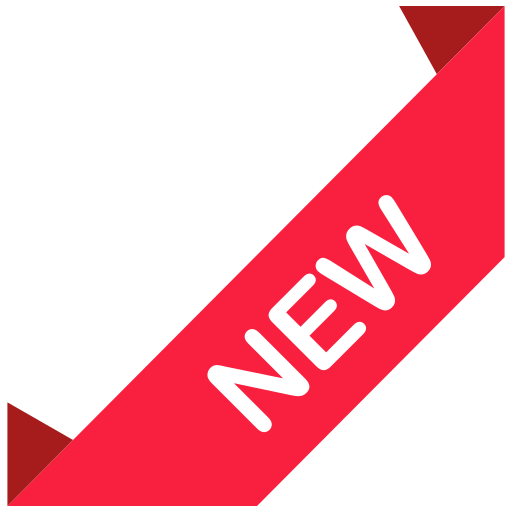
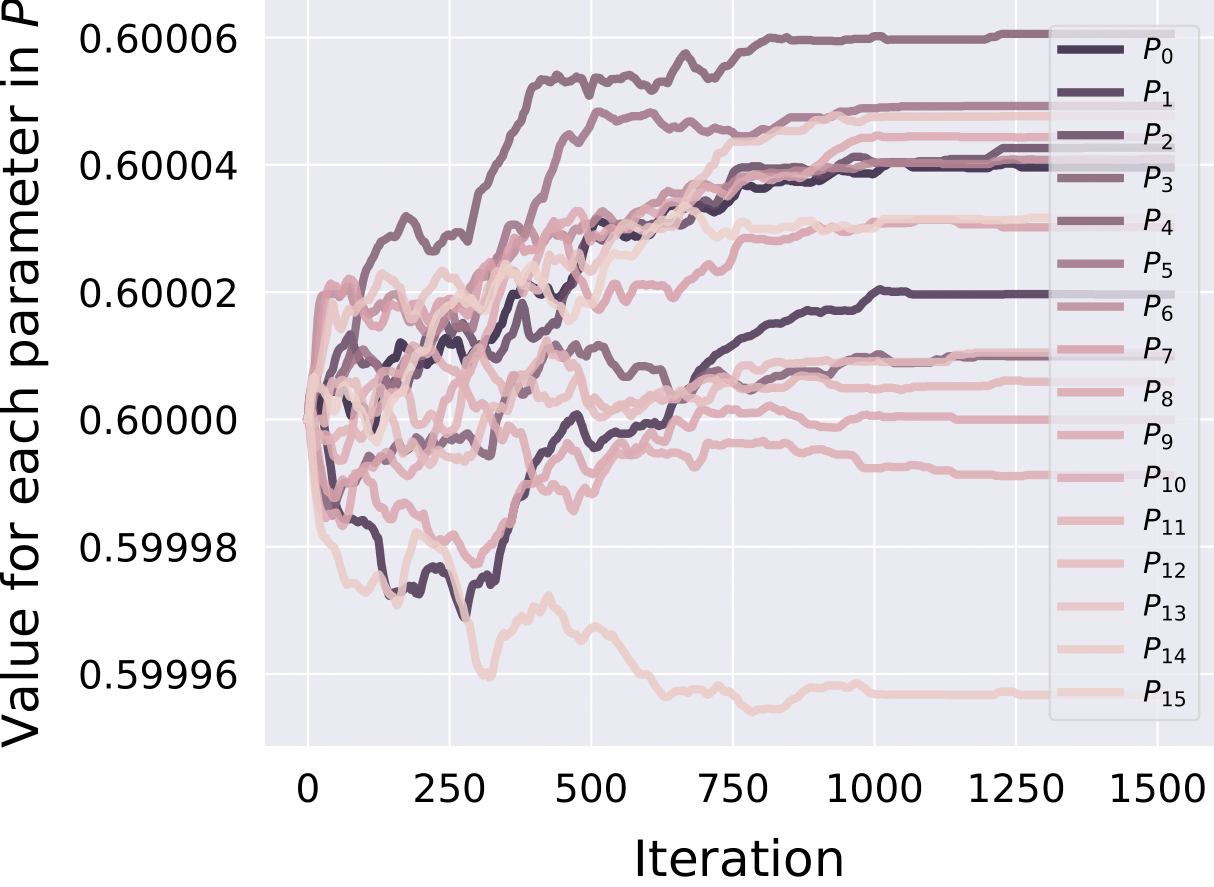
S3 was accepted to NeurIPS 2024!
Posted on: September 26, 2024
🎉 Exciting News! 🎉 Excited to announce that our paper "Segment, Shuffle, and Stitch: A Simple Mechanism for Improving Time-Series Representations" has been accepted to NeurIPS 2024! See post
Publications
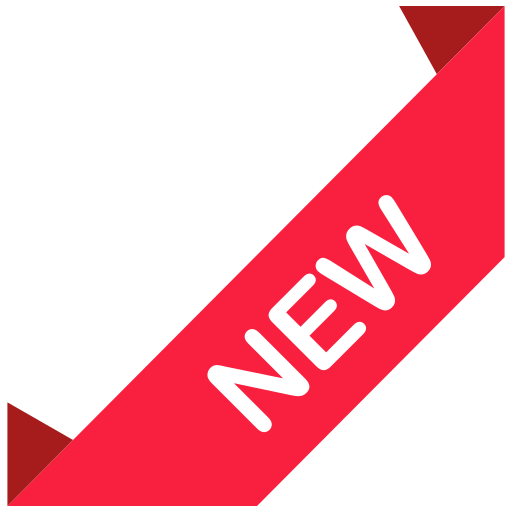
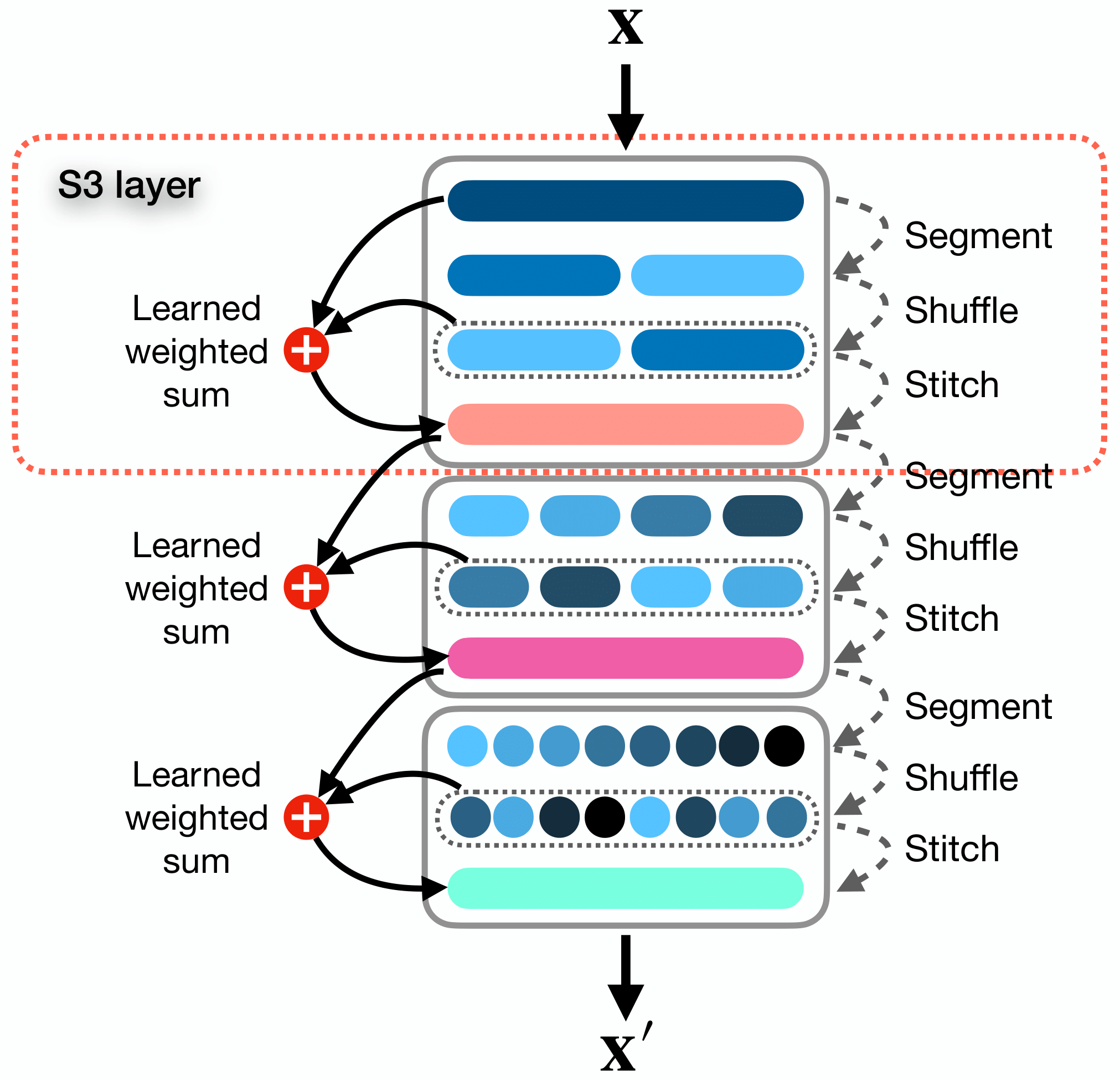
Segment, Shuffle, and Stitch: A Simple Layer for Improving Time-Series Representations
Neural Information Processing Systems (NeurIPS) 2024
Abstract: Existing approaches for learning representations of time-series keep the temporal arrangement of the time-steps intact with the presumption that the original order is the most optimal for learning. However, non-adjacent sections of real-world time-series may have strong dependencies. Accordingly, we raise the question: \textit{Is there an alternative arrangement for time-series which could enable more effective representation learning?} To address this, we propose a simple plug-and-play neural network layer called Segment, Shuffle, and Stitch (S3) designed to improve representation learning in time-series models. S3 works by creating non-overlapping segments from the original sequence and shuffling them in a learned manner that is optimal for the task at hand. It then re-attaches the shuffled segments back together and performs a learned weighted sum with the original input to capture both the newly shuffled sequence along with the original sequence. S3 is modular and can be stacked to achieve different levels of granularity, and can be added to many forms of neural architectures including CNNs or Transformers with negligible computation overhead. Through extensive experiments on several datasets and state-of-the-art baselines, we show that incorporating S3 results in significant improvements for the tasks of time-series classification, forecasting, and anomaly detection, improving performance on certain datasets by up to 68\%. We also show that S3 makes the learning more stable with a smoother training loss curve and loss landscape compared to the original baseline. The code is available at \href{https://github.com/shivam-grover/S3-TimeSeries}{https://github.com/shivam-grover/S3-TimeSeries}.
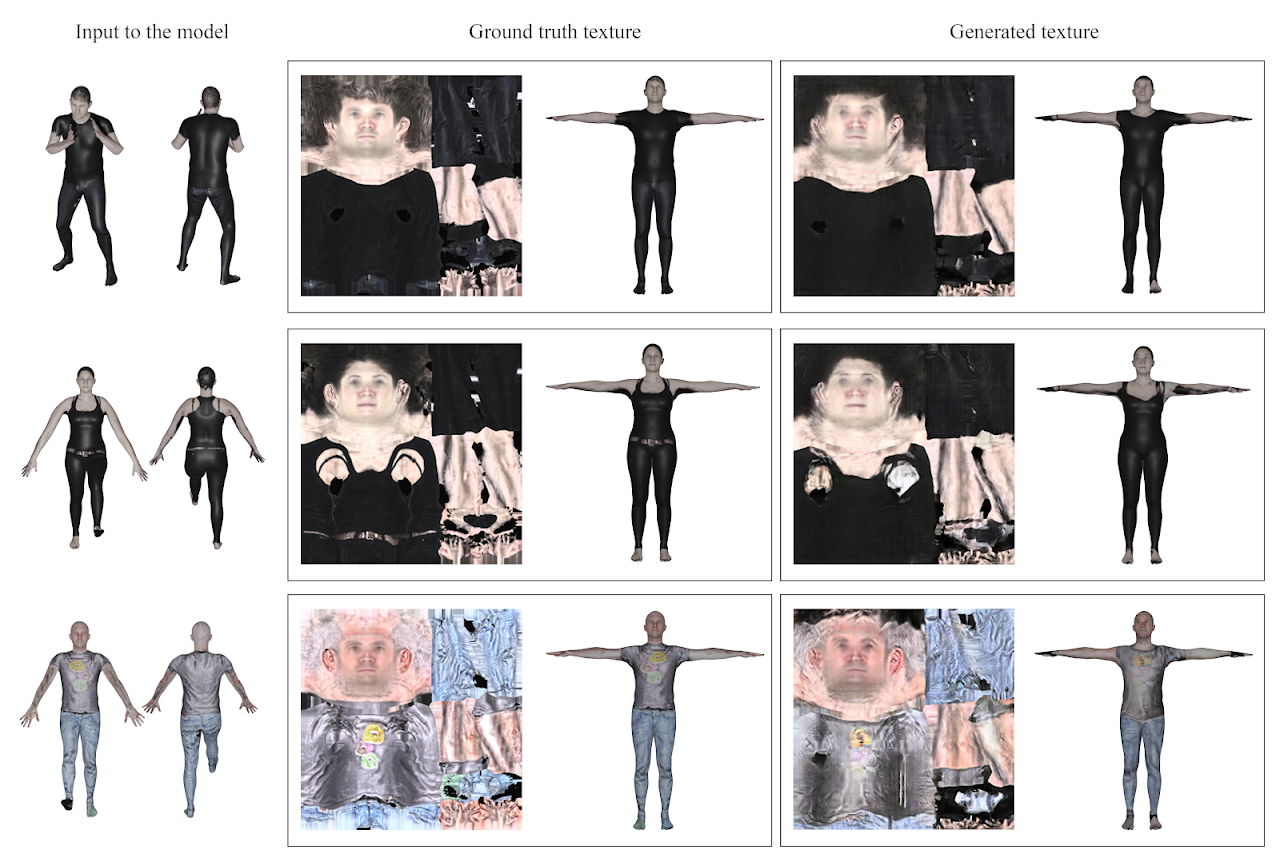
Pipeline for 3D reconstruction of the human body from AR/VR headset mounted egocentric cameras
arXiv preprint
Abstract: In this paper, we propose a novel pipeline for the 3D reconstruction of the full body from egocentric viewpoints. 3-D reconstruction of the human body from egocentric viewpoints is a challenging task as the view is skewed and the body parts farther from the cameras are occluded. One such example is the view from cameras installed below VR headsets. To achieve this task, we first make use of conditional GANs to translate the egocentric views to full body third-person views. This increases the comprehensibility of the image and caters to occlusions. The generated third-person view is further sent through the 3D reconstruction module that generates a 3D mesh of the body. We also train a network that can take the third person full-body view of the subject and generate the texture maps for applying on the mesh. The generated mesh has fairly realistic body proportions and is fully rigged allowing for further applications such as real-time animation and pose transfer in games. This approach can be key to a new domain of mobile human telepresence.